COLUMBUS, OHIO -- TECH CRUNCH -- Defrag, the world’s first, only, and best “Data Platform Data Platform”, today announced that it received $42 million in Series A financing in a round led by _tbd Ventures.
The Defrag platform enables data teams to join scattered data platform metadata and put it to use in other tools. It currently has integrations with 46 tools in the modern data stack. Dozens of data teams use Defrag to coordinate information about data extractions, data workflows, data quality processes, data compliance, and data usage.
“Currently, critical platform metadata hides underneath changing APIs, incompatible data models, and a general lack of purpose,” says Adam Tate, founder of Defrag. “It’s exhausting for any team to handle on their own, and it’s challenging for innovators to build on top of. With Defrag, engineering these pipelines is not only possible, it’s so simple a data scientist could do it.“
Tate says Defrag’s approach is to take the best aspects of customer data platforms such as Segment — “It’s a solved problem,” he claims — and applies them to the modern data stack. These traits include a highly flexible data model, fully-managed connectors with useful opinions, and versatile export and monitoring functionality. The twist is that, instead of data about people, Defrag is fueled by data about data.
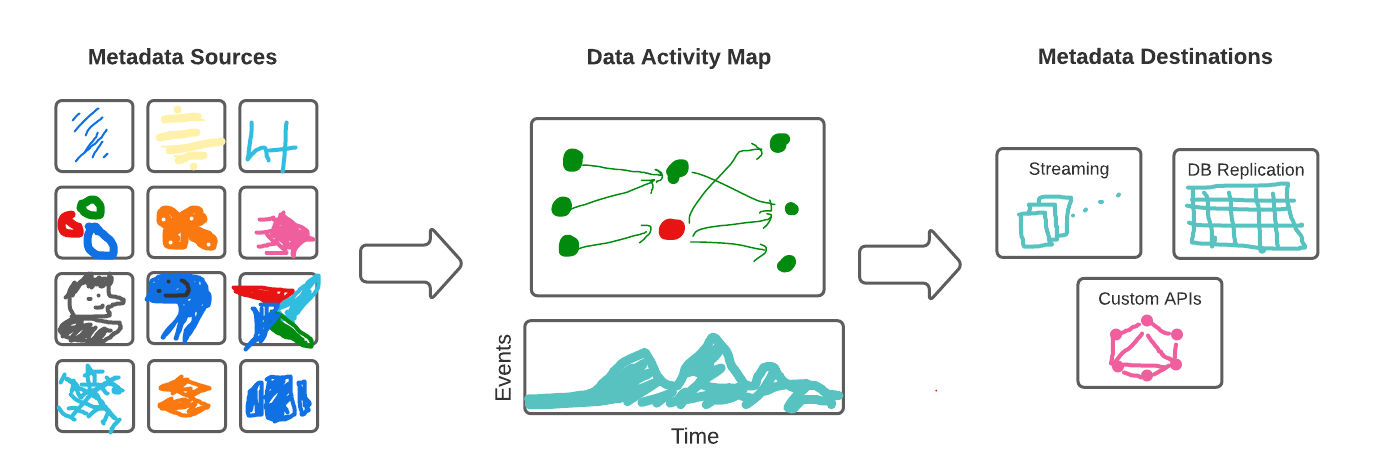
Tate says the product is split into three main components:
Metadata extractors that connect to all layers of the modern data stack, from extraction to consumption to catalog, unlocking metadata use cases across the entire data lifecycle.
A dynamic map of data sources that integrates data sources, lineage, and activity.
Versatile export functionality through specific connectors (e.g. to data catalogs), activity replication back into a warehouse, or the set up of custom API endpoints for ad hoc use cases.
The team currently maintains just under 50 connectors and is planning to add 100 over the next year. “We’re going to have so many logos on our home page,” Tate says, a bit too eagerly. “But that’s the important work to be done right now: we need to get all these systems talking a common language.”
Building a common language for these different systems has been no small feat. Defrag integrates with Tableau dashboards, Airflow DAGs, dbt models, Hightouch syncs, Snowflake query usage, among many others. While all of these operate on data, the specifics vary wildly.
“It’s like a thousand-circle Venn diagram, where every system overlaps each other enough to be relevant, but not enough to be translated easily,” Tate explains. “Most existing tools respect the source system’s opinions, so they create a “DAG” object and a “Dashboard” object and an “ML Model” object. We keep it simple so users can build the tools they want to build.”
“Data Sources” and “Data Actions” are the two main node types in Defrag’s data model. Tate says this model was heavily inspired by Segment, which doesn’t try to model your entire website, for example, except as users touch it. Like established customer data platforms, Defrag developers have access to SDKs that make it easy to write to these tables with simple API calls:
The identify action declares the existence of a data source or updates it. It’s often used during data operations that create new tables, such as a ELT process or dbt run.
The track action is run to log any data action that operates on one or more data sources. These are often events like syncs, tests, and data snapshot processes. Apart from standard “status”, “duration”, “inputs,” and “outputs” attributes, teams can add any additional properties they would like.
Defrag’s off-the-shelf metadata connectors take the pain out of mapping different APIs to this simplified model. After connecting to Fivetran, for example, Defrag will automatically post the identify and track calls at the end of every sync. Tate says they opt to make connectors event-driven wherever possible, but in many cases, regular polling via API is required.
Tate says one of the biggest misconceptions about their product is that it’s an “enterprise data catalog”.
“Catalogs hoard metadata for their own product, so they can charge for more seats. They want to be the “source of truth” about the platform.” Tate is visibly exasperated. “But I just see it as data getting extracted from one system and buried in another. The Defrag stance is: the potential applications of this metadata are endlessly varied. It’s just never been accessible to anyone."
Tate says taking maximum advantage of Defrag requires an “active management” mentality. These teams are as obsessed with tracking activity and usage as they are about the data model itself.
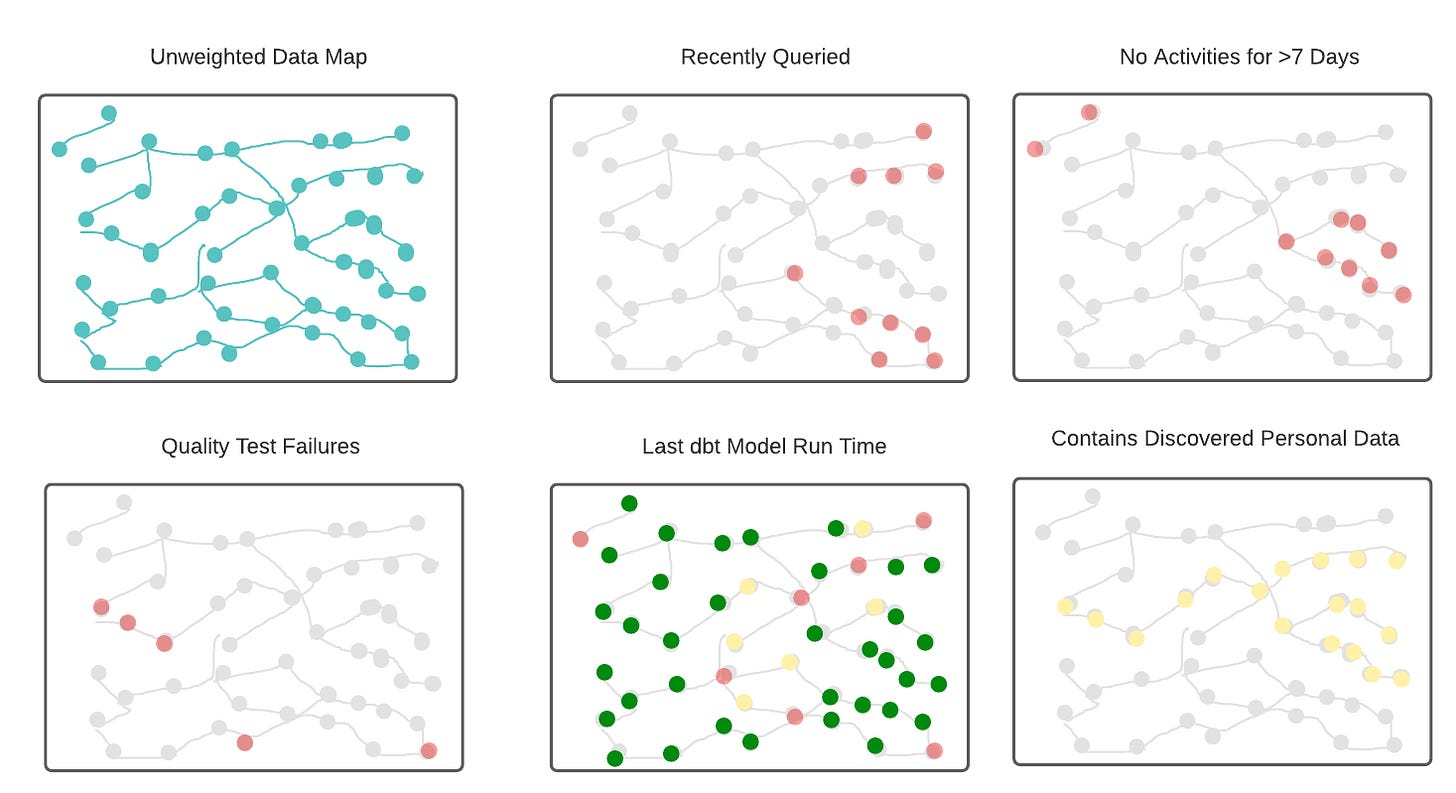
These power users take full advantage of Defrag’s built-in “data activity map”, which combines lineage information with a variety of filter options on both data sources and activities to reveal hotspots in the data platform.
“The first thing I do in the morning is to get some coffee, check our team’s Data Activity Graph. That’s enough to tell me if it’s a ‘get showered and dressed’ kind of day or not,” says data engineer Rod Musselman, a delighted customer of Defrag.
Defrag’s biggest opportunity may lie in what hasn’t been built yet.
“Look, we don’t want to build column-level lineage. We don’t want to build incident management. We don’t want to build a sleek explore experience. We want other people to build that, to build great tools, to make their share of all this data cash,” Tate says. “We want to see metadata put to good use, while also preventing the industry from coming to a halt when dbt Labs upgrades their product to version 2.0, or introduces a new type of node, or modifies their artifacts schema. Defrag can simplify and harden this ecosystem.”
To enable this vision, Defrag has built a library of external connectors that teams can integrate from day one. These come in three forms:
Stream destinations send metadata directly to a tool, such as a data catalog, as it comes into the Defrag platform.
Table replication makes the raw data source and activity data available directly to data teams so that they can build on top of it, build custom reporting, or integrate it with other assets.
Custom API endpoints that will return a payload usable in downstream tools. One common example is returning an HTML response that can be embedded in an iFrame downstream.
As new use cases are uncovered, they are added to the library. One of Defrag’s most popular extensions is also one of its simplest: a widget that consolidates Data Action metadata across a set of data sources.
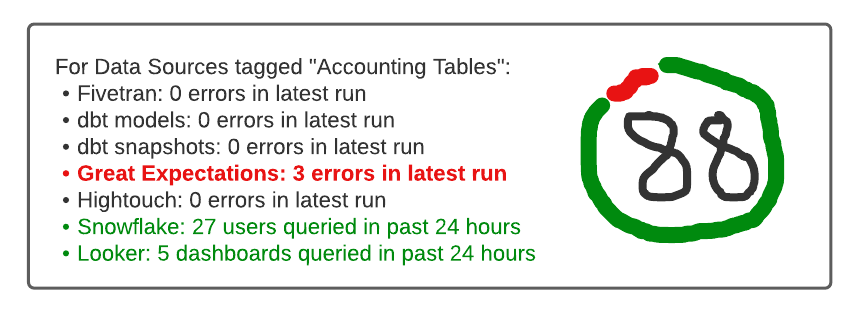
Musselman, the data engineer explains the appeal, “Our users just go bananas for these pie charts on their dashboards. And it’s great for me because I can just update the Data Source tags in dbt and everything downstream gets updated as I expect.”
Although use cases are abundant, Tate is focused on Defrag’s role as facilitator: “My entire existence is literally predicated on simplifying metadata. Defrag will provide value when a team has 10 tables and 50 activities per day. It will provide value when there are 1,000 tables and 5,000 activities per day.”
Tate is in it for the long haul. “No one knows what the data stack will look like in ten years, but I can guarantee you this: metadata will be the glue.”
I have never met a better substack...subscribed and cannot wait for more.
Why isn't this actually a thing? Like, the trapped metadata issue is super duper real...